Diving (back) into Python - Notebooks
21/09/2024
As I've continued to spend more time bringing myself up to speed with Python, I've found myself using notebooks more and more - but with a twist. I'm sure most readers are probably familiar with notebooks by now, but I don't recall them being around when I was using Python heavily last - from memory, iPython was around, but Jupyter hadn't been invented.
Notebooks
For the uninitiated, notebooks were originally pioneered by Mathematica, but came to prominence as part of the Jupyter project (originally iPython Notebook), here's an early description about it from Linux Weekly News (a site I thoroughly recommend by the way, and have been reading since I was in university, which is mumble years ago now).
They work by hosting code (in this case Python) in individual blocks called Cells, that can be executed individually, within an interpreter that keeps running (the Kernel). The code is run in the default Python namespace and so can be accessed as long as the Kernel is running as part of the session. The cells can contain markdown as well as running code. The output from the execution of the cells is written back into the notebook UI, so you can use it a bit like the REPL in Python (or F# or Lisp etc.) The output can be just text from the REPL (e.g. print statements), but can also be visualisations, array output displayed at HTML tables, etc.
This makes Jupyter notebooks into a literate programming environment, with all the affordances of the modern stack. Having the interim state saved and available for inspection can make interactive development a really pleasant experience - and you can document as you go in Markdown so you can express the why as well as the how the code works.
I first started using them in earnest in F# as they support the bottom up functional approach really well and they've become where I start most projects in Python or other languages - they're great for some exploratory programming when you're trying to get an idea up and running, the workflow is to build up short snippets of code in a cell, check it produces the right output, promote it to a function, then build up the program as you go in the same way. When you're in Python, you also have access to libraries such as Matplotlib and Pillow, and so you can see graphs or output of your scripts live in the output cells as you go.
The only real downside is that the structure of the notebook is just a JSON object and because output can change within runs, they don't play very well with Git as virtually any interaction will change the file, so commits can be dirty unless output is cleared.
Notebooks in VSCode
By default, the Jupyter environment is accessed via a browser, but my preferred method is via Visual Studio code (which is where most of my development happens outside of any C# that I happen to be doing). There are extensions available for both Jupyter Notebooks, and also .NET languages such as F# (plus others), called Polyglot Notebooks. The .NET one also supports SQL as a bonus, and can even share data between languages (so, for example, you can execute a query and then plot that in a JS library like ECharts). Another nice feature of Polyglot notebooks is that you can load nuget packages and DLL files from other projects by a magic command. The only real prerequisite is that you need to have Python and Jupyter (to run the kernel) installed for the Jupyter extension, the Polyglot one will require .NET 8.
Here's VSCode running a Python and F# notebook - in this instance, Python was probably the better experience as Matplotlib did the thing I wanted out of the box, whereas I had to try several F# libraries before I got something simple that ran locally.
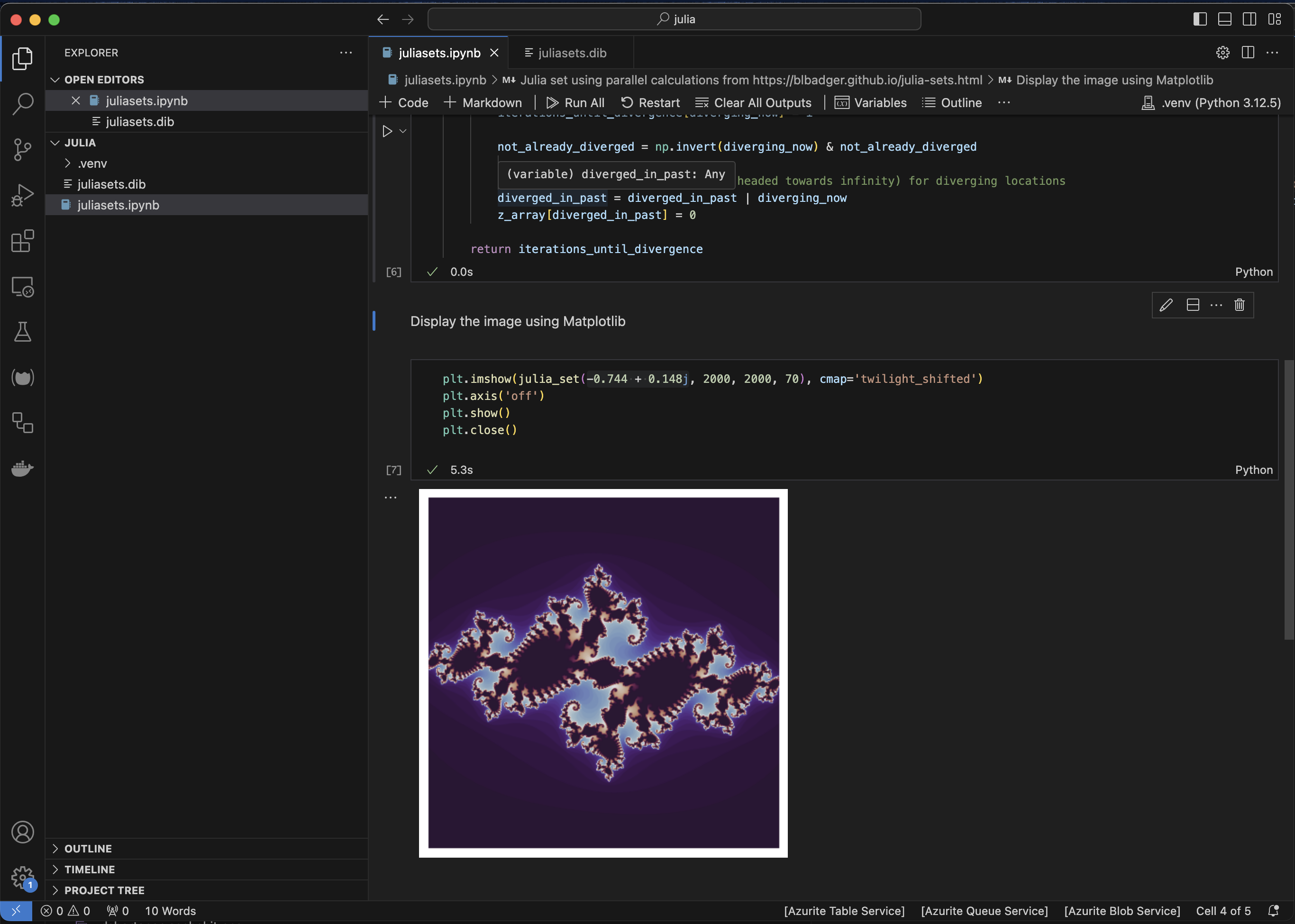
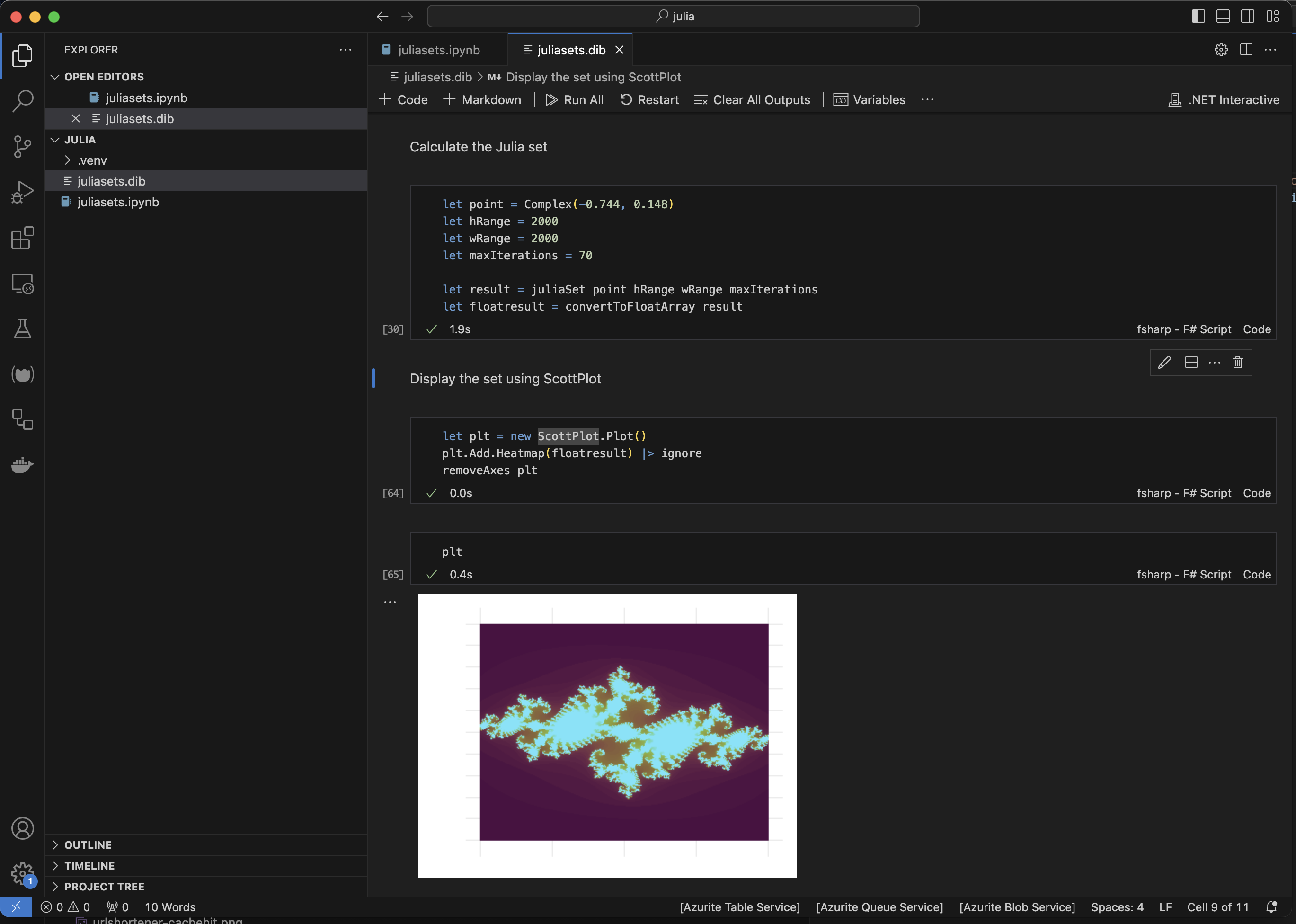
How I use it
As I mentioned earlier, I start a lot of my projects using a notebook, building up short snippets of code that help me flesh out the idea, and then gradually make it more structured (for example, creating functions & modules in F#, or building up classes in Python), and using the markdown documentation to leave a trail of what I did and why, so that I can pick up the experiment a few days or weeks later when I have some free time. Once I've proved the concept then I'll use the nbconvert utility (or the 'Export notebook to script' command in VSCode) to generate a python or F# file that I can continue with generating a normal project. This approach really works for me and it's helped me work productively in fits and starts as time allows.
Bonus - Google Colab
The Google Colab service also uses Jupyer notebooks as its main interface, so I've been using it for some AI related experiments. It's a great place to start as there's a generous free tier of GPU enabled instances, so you have simple access to libraries such as the HuggingFace Transformers library, that makes using most open source large language models very simple to experiment with - more on this in a later post.